
Shrey Khokhra
Sep 13
5 min read
Is AI the Key to Merging Qualitative and Quantitative UX Research for Deeper Insights?
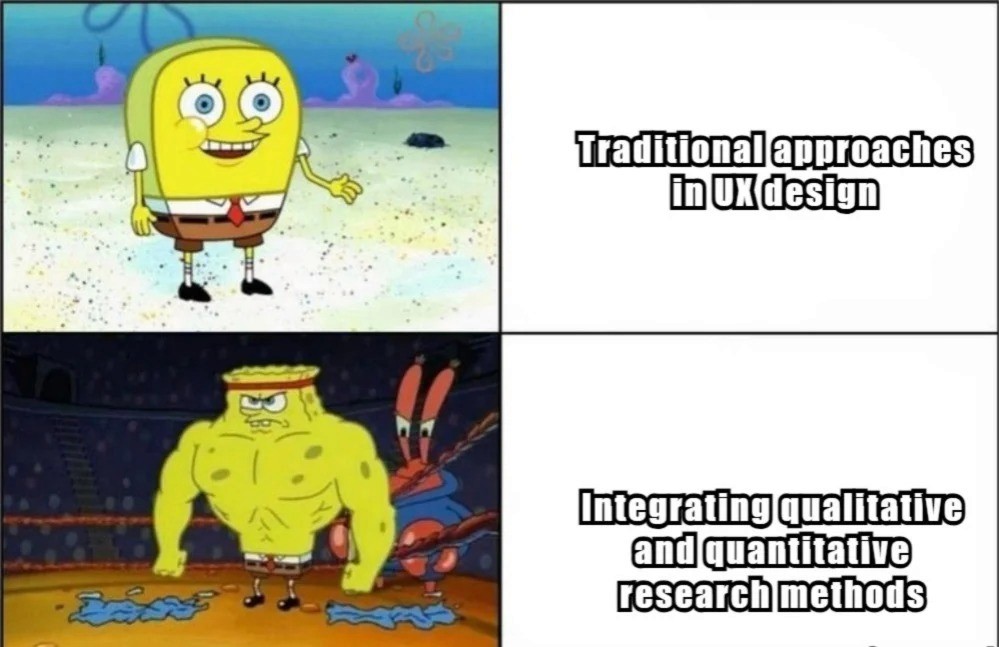
Tl;dr
In the rapidly evolving world of UX design, integrating qualitative and quantitative research methods is essential for gaining a comprehensive understanding of user needs and behaviors. Traditional approaches provide deep insights into user experiences, while quantitative methods offer broader, statistically significant data. However, these methods often face challenges in terms of scale, time, and resource requirements. The advent of AI-powered tools has revolutionised UX research by efficiently merging these methodologies, allowing researchers to simultaneously harness detailed qualitative insights and large-scale quantitative data. By leveraging AI to automate and enhance data collection and analysis, UX research can now achieve both depth and breadth in understanding user behavior. The future of UX research lies in effectively blending these approaches, utilizing AI to create richer, actionable insights while maintaining speed and quality.
The Qualitative Approach: Diving Deep into User Experiences
Qualitative research has long been the cornerstone of UX design. It allows researchers to gain rich, contextual insights into user behaviors, motivations, and pain points. As Peter Drucker aptly puts it:
"The most important thing in communication is hearing what isn't said."
This quote encapsulates the essence of qualitative research – it's about uncovering the unspoken needs and desires of users. Methods like user interviews, usability testing, and ethnographic studies provide invaluable insights that can shape product design and development.
Steve Portigal, a respected UX researcher and author, emphasises:
This deep understanding is crucial for creating user-centred designs. However, qualitative research has its limitations. It's often time-consuming, resource-intensive, and can be subject to researcher bias. Moreover, the small sample sizes typically used in qualitative studies can make it challenging to generalise findings to larger user populations.
The Quantitative Approach: Uncovering Patterns at Scale
On the other side of the spectrum, we have quantitative research. This approach focuses on collecting and analysing numerical data to identify trends and patterns in user behavior. It offers the advantage of larger sample sizes and statistical significance, allowing researchers to make more confident generalisations about user populations.
Jared Spool, a pioneer in UX design and research, notes:
This statement highlights both the strength and limitation of quantitative research. While it excels at answering "what" and "how many" questions, it often falls short in explaining the "why" behind user behaviors.
Quantitative methods like surveys, A/B testing, and analytics can provide valuable insights into user preferences and behaviors at scale. For instance, a study by the Nielsen Norman Group found that increasing sample sizes from 20 to 200 participants in usability studies reduced the margin of error by 65% (Nielsen, 2021). This demonstrates the power of quantitative methods in providing statistically significant results.
However, Jakob Nielsen, co-founder of the Nielsen Norman Group, cautions:
"The problem with quantitative data is that it can be misleading if not properly interpreted."
This emphasizes the need for skilled researchers who can accurately interpret quantitative data and understand its limitations.
The Rise of Mixed-Methods Approaches
Given the strengths and limitations of both qualitative and quantitative methods, many researchers advocate for a mixed-methods approach. Mike Kuniavsky, a respected UX researcher and author, states:
This approach allows researchers to leverage the strengths of both methodologies. For example, quantitative data can identify areas where users are struggling, while qualitative research can delve into the reasons behind these struggles.
Kim Goodwin, another prominent figure in UX research, describes her approach:
This combination of methods can provide a more comprehensive understanding of user behavior and needs. However, implementing a mixed-methods approach traditionally has been challenging due to time and resource constraints. It requires balancing the in-depth, time-consuming nature of qualitative research with the extensive data collection needed for quantitative analysis. Coordinating these two methods can overwhelm research teams, stretching timelines and budgets. Additionally, synthesising findings from both methods can be complex and prone to misinterpretation, as reconciling divergent insights from qualitative and quantitative data isn't always straightforward. This can lead to conflicting conclusions or, worse, skewed insights if one method inadvertently outweighs the other. As a result, many organizations' opt to prioritize one method over the other, sacrificing a holistic view in favor of faster results.
Is Mixed Methods Research Really Efficient in the Age of AI-Moderated Research Tools?
The choice between qualitative and quantitative methods isn't just about methodology—it also has psychological implications. As Christian Rohrer, Vice President of User Experience at Microsoft, explains:
For a complete understanding of user needs, traditional research methods require both a large sample size to identify trends and in-depth conversations to uncover the motivations behind those trends. This is where mixed methods—combining qualitative and quantitative approaches—excel. Mixed methods can offer a comprehensive view of user experiences by integrating numerical data with rich, contextual insights. However, implementing this approach can be challenging. It is notoriously time-consuming and resource-intensive, involving:
Large-scale surveys to gather quantitative data.Usability tests to assess user interactions.Analytics to identify patterns.Comprehensive interviews or observational studies to explore user motivations.
These tasks often lead to logistical hurdles, including the coordination of participant recruitment, scheduling sessions, and synthesizing findings into actionable insights. Balancing both methodologies can result in a fragmented and cumbersome process, making it difficult to derive coherent conclusions.
The AI Revolution in UX Research
Enter artificial intelligence (AI). Recent advancements in AI and machine learning are revolutionising UX research by offering new ways to integrate qualitative and quantitative methods efficiently. AI-powered research tools are now capable of:
Analysing vast amounts of user data to identify patterns and trends.Processing unstructured data from sources like customer reviews, support tickets, and social media posts.Extracting both quantitative metrics and qualitative themes simultaneously.
These AI-driven platforms can conduct real-time analysis, automating the identification of trends and the extraction of underlying motivations. As a result, researchers can obtain statistical significance in their data along with detailed insights into why certain trends emerge.
AI-assisted tools not only save time but also enhance the quality of insights. By automating routine tasks such as data collection, transcription, and initial analysis, AI allows researchers to concentrate on strategic aspects like interpreting findings, testing hypotheses, and making design decisions. AI is increasingly adept at uncovering trends and patterns that might be missed by human researchers, offering deeper insights into user behavior and preferences.
Furthermore, AI-driven platforms can provide predictive insights, helping researchers anticipate future user needs. Techniques like sentiment analysis and machine learning algorithms enable AI to detect shifts in user preferences over time, offering a forward-looking perspective on how products or experiences might need to evolve.
A study published in the ACM Digital Library highlights this shift:
"AI-assisted UX research tools have shown a 40% increase in efficiency compared to traditional methods, while maintaining a 95% correlation with human-derived insights" (Smith et al., 2023).
This demonstrates how AI is transforming mixed methods research from a labor-intensive and fragmented process into a seamless and efficient approach. AI not only makes mixed methods research more feasible but also essential for scaling UX research in this modern age.
Examples of AI-Powered Research Tools
Userology How It Helps: Userology is an AI-driven platform that enhances user research by automating participant recruitment and data analysis. It allows researchers to conduct both qualitative and quantitative studies, providing insights from usability tests and user interviews. Userology leverages AI to analyse data, helping teams quickly gather comprehensive insights into user behaviours and needs.Scaling Research: With Userology, researchers can efficiently scale their efforts without sacrificing the depth of insights. The platform supports asynchronous research, eliminating scheduling conflicts and enabling access to a diverse participant pool. This means researchers can gather a large volume of feedback while still obtaining rich, contextual insights, allowing for a more holistic understanding of user experiences.Outset
How It Helps: Outset utilizes AI to streamline the user research process by aggregating feedback from various sources, including surveys and interviews. It combines qualitative insights with quantitative data, enabling researchers to identify trends and sentiments effectively. This holistic approach helps teams understand user pain points and motivations in depth.
Scaling Research: Outset’s AI capabilities allow for rapid analysis of extensive datasets, making it possible to derive actionable insights quickly. Researchers can conduct multiple studies simultaneously, leveraging both qualitative and quantitative methods without the logistical challenges typically associated with mixed-methods research. This scalability enhances the overall efficiency of the research process.
3. Maze
How It Helps: Maze automates the analysis of user tests and generates reports from both moderated and unmoderated studies. It can process various data types, including video and audio, to extract key findings. This tool provides a seamless way to gain insights from both qualitative feedback and quantitative metrics, enabling researchers to understand user interactions comprehensively.
Scaling Research: Maze's AI-driven analytics facilitate continuous monitoring of user behaviour at scale. By automating data collection and analysis, researchers can handle larger datasets and derive insights more efficiently. This allows teams to focus on interpreting findings rather than getting bogged down in data management, ultimately enhancing the scalability of their research efforts.
4. Loop Panel
How It Helps: Looppanel employs AI to automate the transcription and analysis of user interviews. It organises qualitative data by research questions and uses auto-tagging to help researchers identify key themes and sentiments. This integration of qualitative insights with quantitative metrics allows for a richer understanding of user feedback.
Scaling Research: By automating the analysis of unstructured data, Looppanel significantly reduces the time and resources needed for manual data processing. Researchers can scale their analysis to handle larger datasets, gaining comprehensive insights without increasing team size. This efficiency is crucial for managing the growing complexity of user data in today’s digital landscape.
Impact on Scaling Research
The integration of these AI-powered tools in UX research significantly improves the efficiency and scale of research activities. They automate the collection, analysis, and synthesis of both qualitative and quantitative data, reducing the time and cost associated with traditional research methods. By leveraging AI, researchers can handle larger datasets, gain deeper insights into user behavior, and make data-driven decisions more quickly. This scalability is crucial for keeping pace with the increasing complexity and volume of user data in today's digital landscape.
Addressing Scepticism and Concerns
Despite the promising potential of AI in UX research, many researchers and designers remain sceptical. Common concerns include:
Lack of human touch: Can AI truly understand the nuances of human behavior and emotion?Data privacy: How can we ensure user data is protected when using AI-powered tools?Bias in AI: Could AI algorithms perpetuate or amplify existing biases in research?Overreliance on technology: Will AI tools lead to a decrease in critical thinking and analysis skills among researchers?
These are valid concerns that need to be addressed as the field evolves. It's crucial to remember that AI tools are meant to augment human researchers, not replace them. As Dana Chisnell, a renowned UX researcher, notes:
"I believe that the most effective research methods are those that combine the strengths of both qualitative and quantitative approaches."
AI-powered tools can help achieve this combination more efficiently and at a larger scale than ever before.
The Future of UX Research: A Balanced Approach
As we look to the future, it's clear that the most effective UX research strategies will be those that balance qualitative and quantitative methods, leveraging technology to scale research efforts without losing the human touch.
Nikki Tsizat, a pioneer in remote user research, sums it up well:
This is where innovative solutions like Userology's Nova come into play. Nova is an AI-powered UX research tool designed to bridge the gap between qualitative and quantitative methods. It allows researchers to conduct large-scale studies that capture both numerical data and rich, contextual insights.
With Nova, researchers can:
Automate participant recruitment and screening, ensuring diverse and representative samples.Conduct asynchronous research sessions, eliminating scheduling conflicts and no-shows.Analyse both structured and unstructured data, providing quantitative metrics alongside qualitative themes.Identify patterns and insights that might be missed by human researchers alone.
Most importantly, Nova is designed to complement human researchers, not replace them. It handles the time-consuming tasks of data collection and initial analysis, freeing up researchers to focus on interpretation, strategy, and design implications.
Conclusion: Embracing the Future of UX Research
As the field of UX continues to evolve, so too must our research methods. The future lies not in choosing between qualitative and quantitative approaches, but in finding innovative ways to combine their strengths. AI-powered tools like Nova offer exciting possibilities for scaling UX research without sacrificing depth or quality of insights.
By embracing these new technologies and approaches, we can create more user-centred designs, make more informed decisions, and ultimately deliver better experiences to users around the world. The journey of UX research evolution continues, and the destination looks brighter than ever.